All Common Core: High School - Statistics and Probability Resources
Example Questions
Example Question #151 : High School: Statistics & Probability
Use technology to find the least squares regression equation for the following data set.
In order to solve this problem, we need to define what is meant by the phrase 'least squares regression equation.' Essentially, the least squares regression equation is the equation of the best-fit line of the data if it were graphed on a scatter plot. Trend lines for data that possess a linear relationship are typically written in the slope-intercept form. This form is commonly written using the equation:
In this equation, the variables are represented in the following manner:
Problems associated with this standard require us to use technology such as statistical programs or calculators to find the least squares regression equation. Essentially, we must use technology to calculate the slope and y-intercept of a group of data points. Let's first use an example to illustrate this process. Consider the following data set:
In order to solve this example and calculate the least squares regression equation, we need to insert the data into a spreadsheet program either using copy/paste techniques or manual entry. The data should look like the following figure:
After the data has been entered into the program, we can use formulas to calculate the slope and y-intercept needed for the least squares regression equation. The slope is calculated using the formula outlined in the red box on the following figure. In this equation, the range of cells in column B represents the y-values while cells from column A denote the x-values.
The number one at the end of the equation designates the specific statistic that we wish to calculate. In this case, one means the slope.
We can see that the slope of the least squares regression equation is the following:
Now, let's calculate the y-intercept. It is calculated by using the formula outlined in the red box of the following figure. Again, column B represents the y-values while cells from column A denote the x-values.
The number two at the end of the equation designates the specific statistic that we wish to calculate. In this case, two means the y-intercept.
We can see that the y-intercept of the least squares regression equation is the following:
Now, we can write the least squares regression equation:
We can check this answer by graphing the points on a scatter plot and fitting it with a trendline.
We can see that the answers are the same. Now, we have learned how to find the least squares regression equation using technology. Let's use this information to solve the given problem. When we insert the data into a statistical program we can calculate the following information:
y-intercept=
slope=
Let's input this information into a least squares regression equation in the slope intercept format:
Substitute in our calculated values and solve.
Example Question #152 : High School: Statistics & Probability
Use technology to find the least squares regression equation for the following data set.
In order to solve this problem, we need to define what is meant by the phrase 'least squares regression equation.' Essentially, the least squares regression equation is the equation of the best-fit line of the data if it were graphed on a scatter plot. Trend lines for data that possess a linear relationship are typically written in the slope-intercept form. This form is commonly written using the equation:
In this equation, the variables are represented in the following manner:
Problems associated with this standard require us to use technology such as statistical programs or calculators to find the least squares regression equation. Essentially, we must use technology to calculate the slope and y-intercept of a group of data points. Let's first use an example to illustrate this process. Consider the following data set:
In order to solve this example and calculate the least squares regression equation, we need to insert the data into a spreadsheet program either using copy/paste techniques or manual entry. The data should look like the following figure:
After the data has been entered into the program, we can use formulas to calculate the slope and y-intercept needed for the least squares regression equation. The slope is calculated using the formula outlined in the red box on the following figure. In this equation, the range of cells in column B represents the y-values while cells from column A denote the x-values.
The number one at the end of the equation designates the specific statistic that we wish to calculate. In this case, one means the slope.
We can see that the slope of the least squares regression equation is the following:
Now, let's calculate the y-intercept. It is calculated by using the formula outlined in the red box of the following figure. Again, column B represents the y-values while cells from column A denote the x-values.
The number two at the end of the equation designates the specific statistic that we wish to calculate. In this case, two means the y-intercept.
We can see that the y-intercept of the least squares regression equation is the following:
Now, we can write the least squares regression equation:
We can check this answer by graphing the points on a scatter plot and fitting it with a trendline.
We can see that the answers are the same. Now, we have learned how to find the least squares regression equation using technology. Let's use this information to solve the given problem. When we insert the data into a statistical program we can calculate the following information:
y-intercept=
slope=
Let's input this information into a least squares regression equation in the slope intercept format:
Substitute in our calculated values and solve.
Example Question #153 : High School: Statistics & Probability
Use technology to find the least squares regression equation for the following data set.
In order to solve this problem, we need to define what is meant by the phrase 'least squares regression equation.' Essentially, the least squares regression equation is the equation of the best-fit line of the data if it were graphed on a scatter plot. Trend lines for data that possess a linear relationship are typically written in the slope-intercept form. This form is commonly written using the equation:
In this equation, the variables are represented in the following manner:
Problems associated with this standard require us to use technology such as statistical programs or calculators to find the least squares regression equation. Essentially, we must use technology to calculate the slope and y-intercept of a group of data points. Let's first use an example to illustrate this process. Consider the following data set:
In order to solve this example and calculate the least squares regression equation, we need to insert the data into a spreadsheet program either using copy/paste techniques or manual entry. The data should look like the following figure:
After the data has been entered into the program, we can use formulas to calculate the slope and y-intercept needed for the least squares regression equation. The slope is calculated using the formula outlined in the red box on the following figure. In this equation, the range of cells in column B represents the y-values while cells from column A denote the x-values.
The number one at the end of the equation designates the specific statistic that we wish to calculate. In this case, one means the slope.
We can see that the slope of the least squares regression equation is the following:
Now, let's calculate the y-intercept. It is calculated by using the formula outlined in the red box of the following figure. Again, column B represents the y-values while cells from column A denote the x-values.
The number two at the end of the equation designates the specific statistic that we wish to calculate. In this case, two means the y-intercept.
We can see that the y-intercept of the least squares regression equation is the following:
Now, we can write the least squares regression equation:
We can check this answer by graphing the points on a scatter plot and fitting it with a trendline.
We can see that the answers are the same. Now, we have learned how to find the least squares regression equation using technology. Let's use this information to solve the given problem. When we insert the data into a statistical program we can calculate the following information:
y-intercept=
slope=
Let's input this information into a least squares regression equation in the slope intercept format:
Substitute in our calculated values and solve.
Example Question #11 : Fit A Linear Function For A Scatter Plot: Ccss.Math.Content.Hss Id.B.6c
Use technology to find the least squares regression equation for the following data set.
In order to solve this problem, we need to define what is meant by the phrase "least squares regression equation." Essentially, the least squares regression equation is the equation of the best-fit line of the data if it were graphed on a scatter plot. Trend lines for data that possess a linear relationship are typically written in the slope intercept form. This form is commonly written using the equation:
In this equation, the variables are represented in the following manner:
In order to solve this question and calculate the least squares regression equation, we need to insert the data into a spreadsheet program either using copy/paste techniques or manual entry. The data should look like the following figure.
After the data has been entered into the program, we can use formulas to calculate the slope and y-intercept needed for the least squares regression equation. The slope is calculated using the formula outlined in the red box on the following figure. In this equation the range of cells in column B represents the y-values while cells from column A denote the x-values.
The number one at the end of the equation designates the specific statistic that we wish to calculate. In this case, one means the slope.
We can see that the slope of the least squares regression equation is the following:
Now, let's calculate the y-intercept. It is calculated by using the formula outlined in the red box of the following figure. Again, column B represents the y-values while cells from column A denote the x-values.
The number two at the end of the equation designates the specific statistic that we wish to calculate. In this case, one means the y-intercept.
We can see that the y-intercept of the least squares regression equation is the following:
Now, we can write the least squares regression equation:
We can check this answer by graphing the points on a scatter plot and fitting it with a trendline.
We can see that the answers are the same. Now, we have calculated how to find the least squares regression equation using technology.
Certified Tutor
Certified Tutor
All Common Core: High School - Statistics and Probability Resources
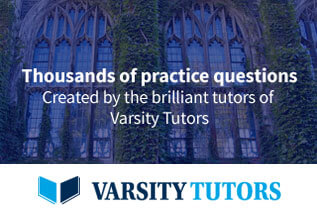